The importance of distinguishing between the odds ratio and the incidence rate ratio in GWAS
Most GWAS are built on unrelated case-control studies, e.g. studies within the Psychiatric Genomics Consortium. In this paper we investigate the difference between the odds ratio, estimated using an unrelated case-control study, and the incidence rate ratio from longitudinal designs. From simulations we see that the odds ratio is not equal to the incidence rate ratio, and that these differences are reflected in the resulting p-values. The odds ratio measures the effect of the SNP on the probability of being diseased, whereas the incidence rate ratio measures the effect on the probability of becoming diseased.
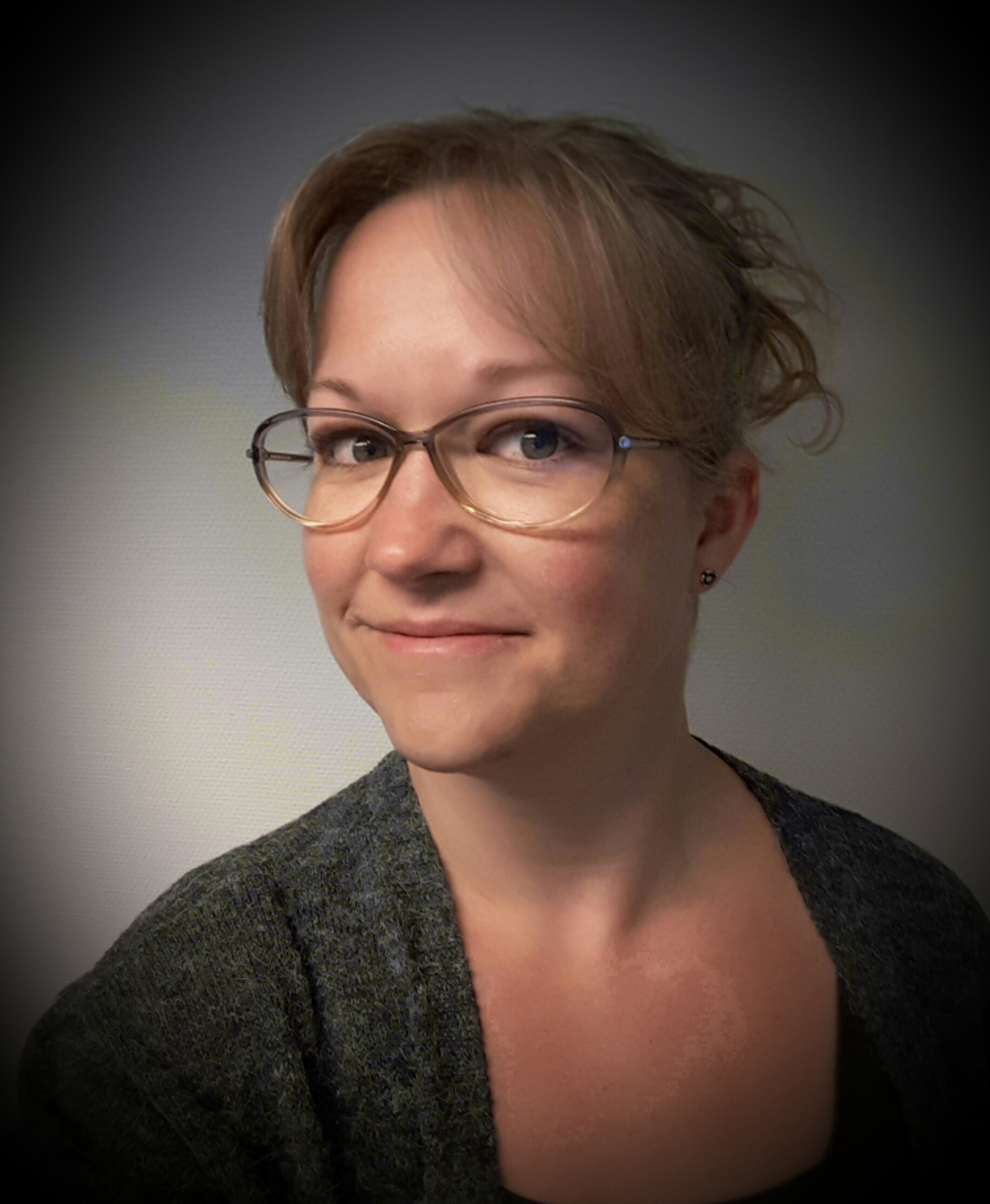
About the study
There is a difference between scientifically asking the question: “does SNP have an effect on the probability of being diseased?” and “does SNP have an effect on the probability of becoming diseased?”. The first hypothesis is investigated using an unrelated case-control study and the odds ratio (OR). The second hypothesis is investigated using longitudinal data, e.g. a matched case-control study comparing controls with the same age as case, when diagnosed. Here the measure of interest is the incidence rate ratio (IRR). The second hypothesis concerns the etiology of the disease of interest; while the first hypothesis does not necessarily, due to competing events, such as individuals dyeing before getting diseased.
In this paper we introduce the effects of competing events and examine the difference of the OR and the IRR in the two different epidemiological studies using simulations. Further the effect of these differences on the p-values is illustrated.
Longitudinal data were simulated using a competing risk model with two events: disease and death. Four different disease-scenarios are studied: A) a common disease with early onset, B) a rare disease with early onset, C) a common disease with later onset, and D) a rare disease with later onset. Different effects of the minor allele count on the IRR of disease and the IRR of death is simulated in a Cox proportional hazard model.
All most all simulated scenarios showed a difference between the estimated OR and the true IRR used for simulation. Both larger and smaller values of OR are observed compared to the true IRR. The only scenario with no difference is rare diseases, where the number of minor alleles is not effecting the IRR of death. This is known as the rare disease assumption. Both false positive and false negative associations can occur when wrongly interpreting the estimated OR or IRR with respect to the two hypothesis described above, i.e. the number of variant alleles may be associated with disease through the OR but not through the IRR or vice versa.
Careful considerations are needed prior to any scientific investigation. In the case of association studies, specification of the hypothesis in question is of high importance even in so-called hypothesis free analyses, e.g. GWAS. Miss-interpretations of the measured effect can be crucial. However, the longitudinal sampling of the iPSYCH cohort provides possibilities for investigating factors associated with becoming diseased.
The article “The importance of distinguishing between the odds ratio and the incidence rate ratio in GWAS” was published in BMC Medical Genetics 2015, 16:71
Facts about the study
- The rare disease assumption is compromised under competing risks.
- The sampling design depends on the hypothesis in question.
- There is a different interpretation of the two measures: the incidence rate ratio and the odds ratio, answering the two questions “is SNP associated with becoming diseased?” and “ is SNP associates with being diseased?”.
- Different epidemiological designs are needed to estimate the incidence rate ratio and the odds ratio.
Further information:
Berit Lindum Waltoft, MSc in Statistics, PhD student, The National Centre for Register-based Research and the Bioinformatics Research Centre, Aarhus University
E-mail: berit@econ.au.dk